The Technical Trajectory from LLM Chats to Agentic Workflows and Physical AI -– Paul Hsu, Founder and CEO at Decasonic
Irrespective of the financial market cycles, we are in the early days of a Web3 and AI supercycle. The technical and product trajectories of this intersection has reached a point of no return, accelerating at an exponential rate, as the permissionless, open source nature of this community innovation compounds. Artificial intelligence is evolving rapidly, moving beyond conversational Large Language Models (LLMs) into autonomous agent workflows and embodied “physical AI” systems.
At Decasonic, we monitor the technical trajectory of disruptive innovations in our next generation internet and align our investments with the accelerating and durable adoption that result from these innovations. I hope that by sharing our insights from an early-stage venture and digital assets investor perspective, we engage with the other investors and innovators at this intersection of Web3 x AI. My goal is to provide early-stage investors and builders with a clear, structured insight into the ecosystem’s trajectory.
We examine technical shifts from LLM-based chat to agentic and robotic AI, key market trends, investment opportunities, strategic positioning for startups and enterprises, and Nvidia’s roadmap implications. This report highlights the current evolution of the AI ecosystem trajectory, and provides relevant insights to future founders and other VCs at this intersection.
1. Technical Trajectory, 2025- 2030: From LLM Chat to Agentic and Physical AI
At Decasonic, we have been sharing our perspectives and research around Web3 and AI since 2023. Our 2023 Web3 and AI Market Map is below
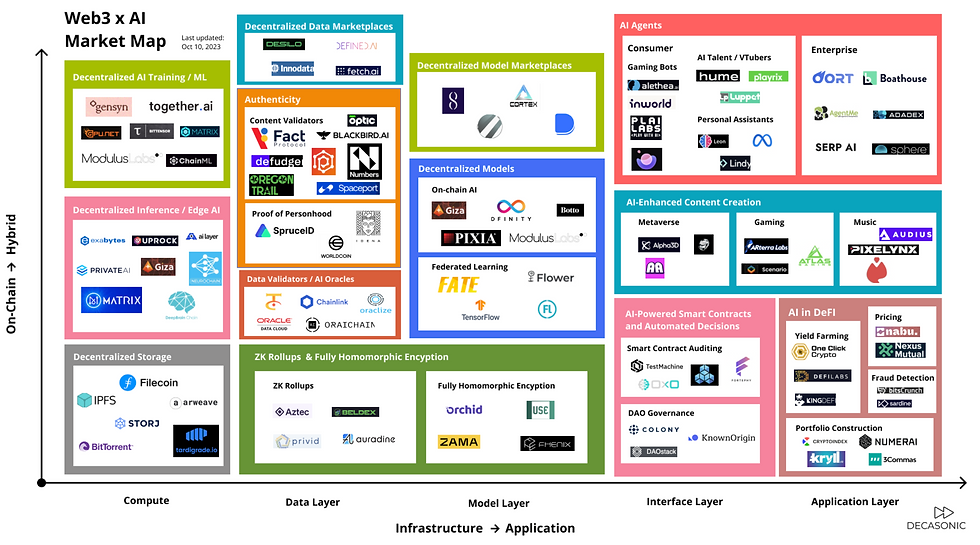
And we have subsequently updated this for 2024.
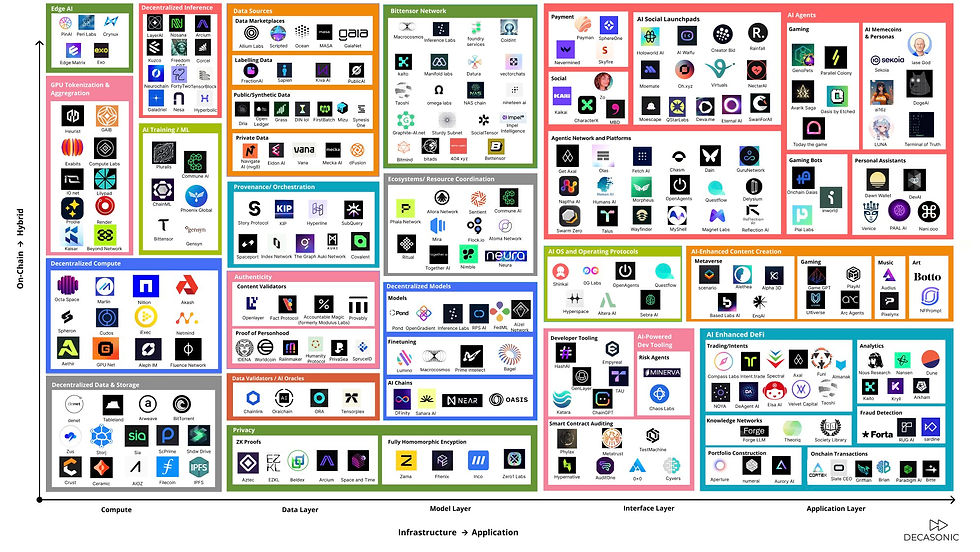
Present Day Evolution of AI Models and Architectures
We are in an era of AI applications and interfaces that will drive and lock in adoption. Mainstream adoption of AI is accelerating, as 400M users access ChatGPT weekly (up 33% since December 2024) with over 2M paying enterprise users (up 100% since September 2024). Importantly, advanced AI adoption is up 5x for Open AI.
AI models are transitioning from standalone LLM chatbots, like ChatGPT, to agentic AI systems capable of autonomous action. The trajectory of LLMs represents enhanced intelligence towards reasoning and eventually general intelligence. The trajectory of chatbots is native and natural interfaces, with engagement lock in through workflows, multiplayer collaboration, and relevant memory for personalization.
Agentic AI refers to AI programs with “agency.” They can plan, make decisions, and adapt with tools independently to perform tasks and complete outcomes for users. Instead of a single LLM answering queries, agentic systems use an LLM to orchestrate multiple specialized agents. This allows dynamic workflows: for instance, an LLM could delegate subtasks to a code-generation model or a search tool, then integrate the results in the digital world. Such multi-agent orchestration marks a shift from simple Q&A bots to AI agents that can reason, iterate, and act in complex environments.
Agentic Workflows and Frameworks
Next-generation AI workflows are increasingly iterative and tool-integrated. Industry research highlights that agentic AI often leverages Retrieval Augmented Generation (RAG) stacks and advanced data architectures . In practice, this means LLMs will be augmented with databases, real-time data access, and domain-specific modules. Frameworks are emerging to support this: for example, libraries that let developers chain LLM calls, manage long-term memory, or integrate with external APIs/IoT devices. These frameworks enable AI agents to perform multi-step tasks (e.g., reviewing a contract, planning a marketing campaign, executing a crypto trade) by consulting knowledge bases and iteratively refining outputs. Over the next few years, we expect rapid improvements in prompt engineering, driven by the continuous integration of AI into various workflows, alongside rapid open-source driven innovation and safety techniques (to keep autonomous agents aligned with user goals). Responsible innovation considers this alignment, intentional or unintentional, with dynamic guardrails in place.
Rise of Physical AI and Embodied Systems
Beyond software agents, AI is becoming embodied in the physical world. At Decasonic, we see the migration from AI in the digital world towards AI in the metaverse (or hybrid world) and AI in the physical world, or Physical AI.
Physical AI refers to AI integrated with robotics and IoT, enabling machines to perceive and act in real environments. A major trend is using simulation and generative models to train robots virtually. Robots can now learn by practicing in simulated worlds (akin to a “Minecraft for robots”) before operating in reality. This approach reduces the need for manual programming by letting robots acquire skills through trial and error in safe virtual spaces. The International Federation of Robotics notes that companies are investing in AI-driven robotics simulation, aiming for a “ChatGPT moment” in robotics. These robots are able to leverage existing Web3 projects, including DePIN devices in order to further acquire a sense of space and physical sense.
LLMs, Agents and Robotics Integration
In parallel, large multimodal models are being applied to robotics. Recent research indicates that LLM-based embodied AI “generalist” robots could become commonplace in homes and industries within a decade. These robots represent an upgrade to today’s specialized robots and would understand natural language instructions, plan complex sequences, and manipulate objects, all guided by foundational AI models. The convergence of LLMs with computer vision and control is enabling robots to understand arbitrary language commands – a big leap from today’s pre-programmed bots.
Over the next 3–5 years, we expect initial deployments of service robots and autonomous vehicles that use advanced AI reasoning to handle unpredictable real-world scenarios. This encompasses everything from warehouse robots that coordinate via AI agents to smart drones and self-driving cars improving through continual learning. The shift is providing a home for agents beyond software, but in the physical realm. IoTeX is one project in Web3 that aims to be the bridge for agentic and physical AI, providing the infrastructure and the tooling through its SDKs and L1 to enable trustless coordination.
Technical Outlook
In summary, the technical trajectory is clear: conversational AI is evolving into autonomous AI agents, and those agents are increasingly gaining a physical form. This will be supported by new model architectures (for reasoning and planning), better frameworks (to connect models with tools and sensors), and hardware optimized for real-time, multi-modal AI processing. The result will be AI systems that are more proactive, context-aware, and capable of end-to-end task execution, whether purely in software or in the physical world.
2. Market Trends: Adoption Dynamics and Influencing Factors
Our 2025 Outlook
We also shared our 2025 Outlook, reflecting the early days of this Web3 x AI supercycle.
1- Blockchain transforms the economics of AI infrastructure, driving advantages for open source AI.
Decentralized compute platforms are reshaping AI infrastructure by providing scalable, cost-efficient alternatives to centralized providers. These platforms democratize access to the massive compute power and costs needed to deploy AI models.
By leveraging blockchain’s programmability and transparency, developers can significantly reduce costs while ensuring efficient resource utilization. Blockchain-powered platforms for verified data and AI models also align digital ownership and incentives between contributors and developers.
Transparent systems enable high-quality datasets to flow seamlessly to AI developers while rewarding contributors through clear, on-chain incentives. This collaborative ecosystem marks a new era for open-source AI—one that is composable, interoperable, and scalable.
2- Web3 tokens crowdfund permissionless AI innovation, powering community innovations.
Web3 tokens are aligning crypto and AI innovation through decentralized funding and governance. AI agent launchpads, powered by token economies, enable communities to crowdfund and deploy autonomous AI agents across diverse decentralized applications.
These agents optimize blockchain transactions, automate workflows on digital platforms, and unlock new possibilities within DeFi, gaming, and decentralized governance. Sophisticated token designs further align economic incentives among developers, AI agents, and users, fostering a virtuous cycle where adoption fuels innovation, and innovation accelerates adoption.
3- AI personalizes our digital and physical worlds, enabling new interfaces for the metaverse.
AI-driven personalization is redefining digital and physical interactions, creating highly adaptive experiences that seamlessly integrate human and machine interactions.
Autonomous AI agents are revolutionizing social platforms by enabling AI-to-AI-to-Human relationships, elevating personalization and intelligent automation in communities. AI-powered storytelling, generative multimedia, and intelligent bots are transforming content creation, making virtual environments more engaging and tailored to individual preferences.
These innovations blur the lines between physical and digital realities, with devices like AI-enabled smart glasses from Meta and Ray-Ban marking the first steps toward this future.
4- AI injects advanced reasoning intelligence into blockchain, upgrading Web3’s use cases.
AI is upgrading blockchain’s capabilities by embedding advanced reasoning and decision-making intelligence into decentralized systems. AI-first interfaces, optimized for voice, gesture, and natural conversation, simplify user experiences and lower barriers to Web3 adoption.
Autonomous AI agents are scaling decentralized ecosystems, managing complex processes within DAOs, Web3 games, and DeFi protocols. These agents optimize decision-making, enhance efficiency, and unlock new use cases, from protocol automation to intelligent asset management.
Moreover, AI drives hyper-personalization by delivering tailored workflows, intelligent recommendations, and actionable insights at scale. The Web3 and AI super cycle is a transformative moment for the Internet itself. By integrating decentralized economic systems with intelligent automation, we are creating a more interconnected, efficient, and equitable digital economy. AI agents are enabling new ways to create and manage value, while AI-first applications are driving adoption across industries and use cases. Blockchains provide the infrastructure for transparency, tokenization, and ownership, while AI unlocks intelligence, automation, and personalization.
Generative AI’s Breakout and Agentic Shift
The past two years saw explosive interest in generative AI, with enterprises integrating LLMs into workflows at unprecedented rates. In 2024, global enterprise AI spending was estimated at $307 billion and is forecast to double to $632 billion by 2028 . This indicates a sustained ~29% CAGR as AI crosses from experimentation into mainstream use.
However, not all companies move at the same speed. Initially, many organizations stuck to test and learn consulting, “innovation” pilots or sandboxed projects. Now cautious optimism is turning into broader adoption as success stories emerge as AI capabilities mature and deliver ROI. The next wave – agentic AI – is on the horizon, promising even greater productivity gains. This suggests that organizations are preparing to go beyond chatbots to more autonomous AI-driven processes.
Enterprise Adoption and Industry Uptake
Across industries, AI adoption rates are growing steadily. According to global surveys, around 78% of organizations were using AI in at least one function by early 2024 (up from ~55% just a couple of years prior, showing rapid uptake). Key developments fueling this trend include improved AI model accuracy, more user-friendly tools (APIs, cloud AI services), and proven ROI in areas like customer service, marketing, and operations.
Workforce augmentation is a notable theme: Companies could “double” their effective workforce with digital AI workers (agents) taking on routine tasks that enhance human capbilities. These AI agents will transform speed-to-market, customer interactions, and product design by working alongside human teams. Early evidence of this is seen in how generative AI tools have cut software development times or how AI assistants handle support tickets, freeing humans for higher-value work.
Web3 and AI Convergence
In Web3, AI is poised to play a pivotal role in decentralized applications (dApps) and blockchain networks. One emerging narrative for 2025 is the integration of AI agents into the Web3 ecosystem, extending beyond DeFi into gaming, supply chains, and more. At Decasonic, we have published a number of market maps of companies operating at the intersection of AI: AI x DeFi, AI x GameFi, AI x SocialFi, and AI x RWA amongst other AI related perspectives on our website.
These specialized on-chain AI agents can understand user intent and execute complex sequences (like multi-step DeFi trades, research AI agent bots, or automated NFT marketplace actions) autonomously, optimizing results by adapting strategies in real time. We’re also seeing synergy in Decentralized Physical Infrastructure Networks (DePIN) – where tokenized physical devices (sensors, drones, IoT) contribute to blockchain networks and AI data. AI can intelligently operate these devices and manage data, while Web3 tokens provide economic ownership and related incentives. This convergence could accelerate in the next few years, as projects realize the benefits of combining AI’s automation with blockchain’s trustless coordination and tokenization, which enhances distribution.
Headwinds and Tailwinds
Several factors will shape the pace of AI advancement:
Hardware & Compute: The availability of powerful yet cost-efficient computing (GPUs, TPUs, edge chips) is critical. As AI models grow, the need for efficient inference (not just training) becomes vital. If NVIDIA and others continue to deliver 5-50x improvements in performance per dollar through new architectures, it will lower barriers for deploying complex AI systems (like multi-agent workflows and LLM inference costs) broadly. Conversely, hardware bottlenecks or supply chain issues could slow adoption. Edge AI and AI smart devices may lack the form factor for personalized experiences, but we are encouraged to see how Apple Intelligence and Gemini impact iOS and Android respectively.
Regulation & Trust: Governments are drafting AI regulations around data privacy, model transparency, and safety. Regions with balanced, clear frameworks may see faster enterprise adoption (as companies feel more confident deploying AI at scale), whereas heavy-handed or fragmented regulations could introduce friction. Additionally, building trust in AI – ensuring ethical use, mitigating bias, and preventing misuse – is crucial for widespread acceptance. Many firms are actively managing GenAI risks (inaccuracy, hallucinations, trade secret confidentiality, IP leakage, etc.) and establishing governance (C-Level or board oversight on AI, Web3 and data strategy, much akin to the US national executive branch).
Talent & Ecosystems: The availability of skilled AI talent and robust ecosystems (open-source libraries, research communities, cloud services) can accelerate innovation. Open source innovation including Deepseek, Alibaba Qwen, and Manus AI is rapidly advancing the collective AI ecosystem and pushing the industry forward. If talent gaps persist or companies struggle to integrate AI due to skill shortages, adoption might slow. However, the rise of AI itself is enabling tools that make implementing AI easier (autoML, code generation), which could democratize development. We are also encouraged by the rapid acceptance of crypto and AI tooling by GenZ.
Macroeconomic Climate: Macro conditions (tech public markets, VC funding climate, crypto liquid markets, AI infrastructure spending, enterprise IT budgets, end user consumer demand) will influence how aggressively organizations invest in new AI initiatives. The promise of productivity gains and cost savings with AI might drive continued investment even in tighter conditions, but very risky moonshot projects could face scrutiny if markets tighten. The focus of investors and funds will increasingly shift to the capital efficiency opportunities offered by AI models and companies. Overall, AI is increasingly seen as a strategic necessity. Companies that pull ahead now are expected to build lasting advantages.
In summary, market trends point to accelerating AI integration across sectors, with agentic and physical AI poised to be the next frontier. Yet, successful adoption will depend on supportive infrastructure, thoughtful governance, and clear demonstrations of value. This brings me to the leading platform providing that infrastructure – NVDIA.
3. NVIDIA’s Roadmap and Its Influence on AI Adoption
Hardware Advances Driving AI Evolution
NVIDIA’s trajectory in the next few years will significantly shape both software and hardware facets of Web3 and AI adoption. CEO Jensen Huang has articulated that “the next wave of AI is here,” centered on robotics and physical AI, which will “revolutionize industries”. Concretely, NVIDIA’s GPU roadmap (e.g., the Hopper and upcoming Blackwell architectures) is focused on delivering the massive compute needed for advanced AI: from giant LLMs to multi-agent systems and robotic vision. Relevant to the evolution is the roadmap highlighted by Huang below, noting the shift from Agentic AI to Physical AI, providing a space for agents to live beyond the physical realm.
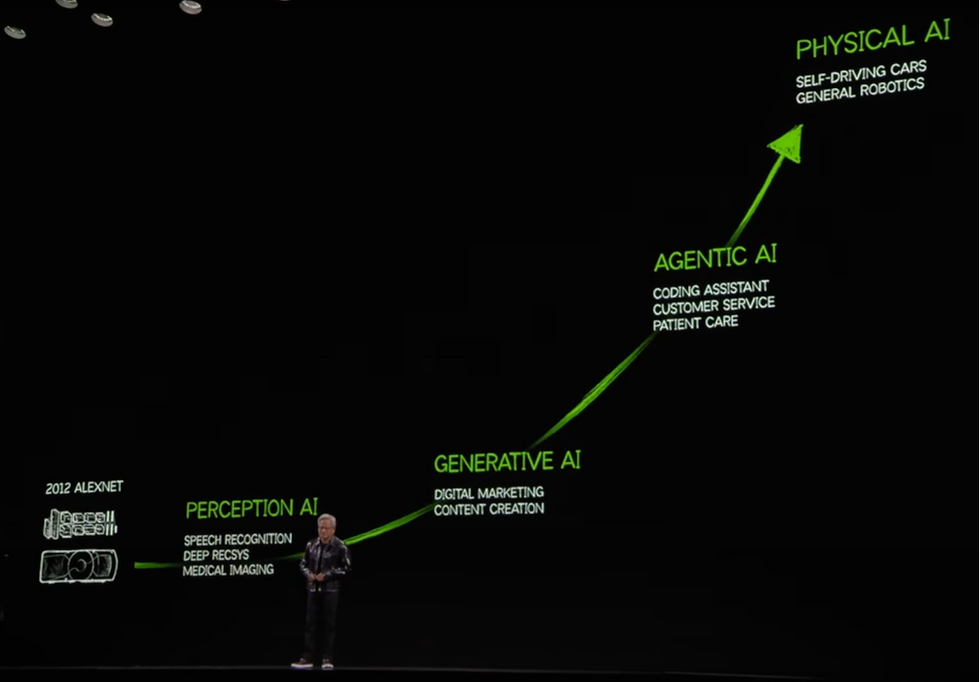
By 2025, inference (deploying trained models in real time) is as critical as training. NVIDIA experts predict that agentic AI’s rise will require near-instant inference across complex multi-model systems . This is pushing NVIDIA to innovate in high-throughput, low-latency hardware – such as the H100/H200 data center GPUs with fast memory, and specialized AI chips for edge devices (Jetson for robotics, DRIVE for autonomous vehicles). Each new generation (often boasting 5–10× performance gains on AI workloads) lowers cost-per-operation, effectively making advanced AI more accessible and scalable.
NVIDIA’s Software Ecosystem
Beyond chips, NVIDIA invests heavily in software stacks that accelerate AI adoption. Their CUDA libraries, AI frameworks, and tools like TensorRT for optimization help developers squeeze maximum performance from hardware. Importantly, NVIDIA is championing simulation and robotics software (e.g., NVIDIA Isaac Sim and Omniverse). These platforms allow training and testing of physical AI in photorealistic virtual environments – aligning with the “Physical AI” trend of robots learning in simulation.
By providing ready-made digital twins and physics engines, NVIDIA shortens development cycles for robotics startups and researchers. We can expect NVIDIA’s roadmap to further integrate AI and graphics simulation, enabling more sophisticated training of autonomous agents (for example, virtual cities to train self-driving AIs, or simulated factories for robot learning). This will accelerate the timeline for getting physical AI from prototype to production, as more scenarios can be ironed out virtually.
Influence on Industry and Startups
NVIDIA’s dominance in AI compute also shapes market behavior. When NVIDIA releases a new GPU generation or software update, it often unlocks new model capabilities (for instance, larger models or more detailed simulations that were previously infeasible). This can lead to rapid industry shifts: companies that were experimenting with an AI suddenly find they can deploy it at scale because the hardware now supports it.
For startups, aligning with NVIDIA’s ecosystem can be a strategic boon – being early adopters of NVIDIA tech (like using new inference chips or joining their Inception startup program) can yield performance advantages. It’s also notable that NVIDIA’s vision often signals credible future directions: their 2025 prediction highlights humanoid robots and AI agents as key trends, which suggests NVIDIA will invest to support those (perhaps specialized chips for humanoid robotics, or middleware for agent communication).
Competition and Collaboration
While NVIDIA leads, others (AMD, Google TPUs, emerging AI chip startups) are also in the race. For AI builders and investors, a close eye on NVIDIA’s roadmap helps anticipate where mainstream support will be strongest. For example, if NVIDIA emphasizes multi-modal AI (vision and language together), they might integrate that into their libraries, making it easier for developers to build such models – this could influence what kinds of applications flourish.
Additionally, NVIDIA’s moves toward more open software (e.g., contributing to PyTorch or supporting interoperable formats like OpenAI’s Triton) could reduce lock-in and benefit the broader industry. On the hardware side, any supply constraints or pricing changes in NVIDIA’s lineup can affect AI cloud costs, which trickles down to AI startups’ burn rates and enterprise project budgets. NVIDIA’s ability to meet surging demand (or not) for AI GPUs in the next few years will directly impact how fast AI projects can scale across the world.
Accelerating the Adoption Curve
In summary, NVIDIA serves as both a catalyst and a barometer for AI’s progress. Their cutting-edge GPUs and software shorten the time to train enormous models and deploy complex agentic systems, effectively pushing the frontier of what AI can do in a given year. Jensen Huang’s keynote statements and NVIDIA’s product launches often precede leaps in real-world applications – for instance, specialized hardware for real-time speech AI led to more conversational agents in services.
As the world moves from chatbots to full-fledged autonomous workflows and robots, NVIDIA’s roadmap suggests an infrastructure ready to support that evolution. This bodes well for faster adoption: what might have taken a decade could happen in a half-decade when the necessary compute and tools are readily available. For investors and builders, leveraging NVIDIA’s ecosystem (and staying adaptive to its advances) will be key to riding the AI wave successfully.
4. Investment Opportunities in the Emerging AI Landscape
Early-Stage Opportunities
For venture investors, the shift to agentic and physical AI opens new frontiers for innovation. Earlier in this article, I shared our Web3 x AI market maps. We consider how leading companies may create new market categories on the frontier, through a systematic process of aligning narrative, product market fit/ token community fit and execution.
Broadly, consider some of the key areas:
AI Agent Platforms & Applications: Startups building platforms for orchestrating AI agents (think “operating systems” for multiple AIs) are attractive. This could be in enterprise workflow automation, personal assistants, or domain-specific agents (finance, healthcare, etc.). Applications and interfaces that leverage autonomous agents to deliver tangible ROI (e.g., a sales agent that autonomously researches and emails leads, or a crypto trading agent optimizing yield strategies) can gain rapid adoption. Increasingly, advances in platforms dedicated for launching agent applications are providing a lower cost of access, and the moat is shifting towards agents that offer utility and a differentiated edge.
Physical AI and Robotics Integration: With robotics getting smarter, there are opportunities in AI-driven hardware. This spans autonomous drones, warehouse robots, delivery bots, and home assistant robots. Early-stage bets could focus on companies building robotics kits and software that incorporate state-of-the-art AI (vision, language understanding, decision-making). For example, startups extending LLMs to control factory robots, or those offering simulation environments (akin to game engines) to train physical AI at scale. Given projections of a billion+ AI-enabled robots in the next decade, the upside in this category is enormous if technical hurdles are overcome.
AI & Web3 Consumer Applications: In the Web3 realm, AI-powered decentralized apps present a novel investment theme. Projects that use AI to enhance blockchain services – e.g., decentralized AI networks that crowdsource model training (rewarding participants with tokens), or AI agents that automate interactions across DeFi, NFTs, and gaming are a focus area for many investors.The concept of DeAI (decentralized AI) is gaining attention, aiming to distribute AI computation via blockchain for transparency, distribution, and verifiability.
AI x RWA: Additionally, DePIN projects (decentralized physical infrastructure) that blend IoT hardware, AI analytics, and token incentives could create new markets (think decentralized smart grids or sensor networks where AI optimizes operations and blockchain handles value exchange). Early investment in these intersections could Offer founders and builders at this intersection the opportunity to advance and innovate in a new frontier.
AI Infrastructure & Chips: The demand for AI compute isn’t slowing. Beyond established players like NVIDIA, there’s room for upstarts in specialized AI hardware – for example, chips optimized for running multiple agents concurrently or low-power AI accelerators for edge devices (robots, AR glasses, vehicles). Cloud infrastructure tailored to agentic AI workloads (which may require fast cross-communication between models) is another niche – e.g., startups providing high-speed interconnects or memory architectures to support AI agents working in parallel. Also, services that offer AI model hubs, prompt libraries, or safety auditing tools for autonomous systems can become critical parts of the ecosystem.
Novel Interfaces and UX: As AI becomes more pervasive, how humans interact with AI is a ripe area for innovation. This could be multimodal interfaces – voice, AR/VR, haptic feedback for robots – that make agentic and physical AI more intuitive. Startups might create voice assistants that control complex agent workflows (beyond simple commands), or AR applications where an AI overlays guidance in real time (for training workers or enhancing decision-making). Investments in companies that bridge the UI/UX gap – making AI feel more like a collaborator than a tool – could pay off as adoption broadens. Notably, NVIDIA experts predict AI interactions will shift from turn-based query/response to fluid, human-like conversations, which means interfaces with emotional intelligence and personality might become standard . Funding teams working on these “AI personality” or adaptive UI problems is an investment in the next phase of user experience.
Timing and Risks
Investors should align bets with the maturity curve of these technologies. Pure software agent platforms might scale quickly (low marginal costs, viral enterprise adoption), whereas robotics startups will have longer hardware development cycles. Risk factors include technical uncertainty (can the startup truly deliver a working product in these cutting-edge domains?) and competition from big tech (who are also eyeing agent platforms and robotics).
However, large incumbents often leave niches unfilled – for example, a specialized agent for crypto compliance or a robot for a specific industry task – where startups can build strong early traction. Look for defensible tech (patents, deep expertise) and evidence of an ecosystem forming around the product (traction, developer growth, and projects joining the respective ecosystem through integration). Those signals indicate the startup can become a critical piece in the AI value chain. Web3 x AI is a large opportunity, and yet, it remains non-consensus among AI and crypto investors.
5. Strategic Positioning: Gaining an Edge with AI Ecosystem Moats
AI Integration as a Competitive Differentiator
For startups and enterprises alike, simply using AI is no longer a differentiator. AI capabilities are at the forefront of how markets will be created, won or lost. AI first companies have and will sustain greater capital efficient growth than legacy competitors.
It comes down to how you use AI that matters. As many companies adopt off-the-shelf LLMs and tools (which are widely available), competitive edge comes from proprietary integrations and data. An effective strategy is to embed AI deeply into one’s product or operations in a way that’s hard for others to replicate.
For instance, a startup might train domain-specific models on unique user data it gathers, yielding smarter AI agents than any generic model could offer. Enterprises can integrate AI across their value chain – from supply chain optimization to personalized customer experiences – creating a systemic advantage.
The specific choice of LLM is less important than leveraging AI with your institutional knowledge and proprietary data to set yourself apart. In practice, this means building feedback loops where your AI gets better as you serve more customers (hence raising the barrier for new entrants).
Building Ecosystem Moats
One way to solidify an advantage is by developing an ecosystem around your AI solutions. This could involve APIs, developer platforms, or marketplaces that position your service at the center of many third-party applications. For example, if your company provides an AI agent platform, encourage other products to plug into it; as more tools integrate, your platform becomes the go-to hub (akin to how app stores or cloud platforms created moats).
In Web3, a protocol that becomes the backbone for AI-driven transactions or DePIN networks can achieve a network effect where its utility grows as more participants join. Founders should think beyond individual features and consider ecosystem partnerships: being the platform where various stakeholders (developers, businesses, end-users) converge with AI at the core. This can create a virtuous cycle of data and adoption that is self-reinforcing, alongside the opportunity for your project to be at the center of the interconnected AI ecosystem.
Applications and Interfaces as Moats
Sometimes the interface (a magical integration of hardware device, screen and application) is the moat. If a startup creates a highly intuitive application of AI – say a seamless AR assistant for surgeons, Meta Ray ban glasses and other heads up displays, or a financial planning AI with a delightful chat interface – the user experience itself can be a differentiator. Superior UI/UX, trust (through responsible AI and transparency), and first-mover advantage in capturing mindshare are strategic assets. Additionally, owning the distribution channel for AI (e.g., being integrated into popular software suites, or embedded in widely used hardware) provides defensibility.
As AI agents proliferate, we may see companies establishing themselves as the “agent provider” within certain verticals (like an AI agent specialized for legal research that becomes industry-standard, complete with compliance and citation capabilities). These application-layer plays, combined with underlying AI tech, create a full-stack advantage that’s hard to dislodge.
Organizational Readiness and Moat Maintenance
To leverage AI for a competitive edge, organizations must also adapt internally. This means new roles and processes: for example, “AI agent supervisors” or teams responsible for integrating and monitoring AI agents in workflows. Early adopters might create “Centers for AI Agents” (akin to today’s Centers of Excellence) to continuously develop proprietary agents in-house, rather than relying purely on vendors. While that requires upfront investment, it builds know-how that competitors who just buy AI services might lack. Over time, the blended workforce of humans plus AI needs careful management – companies that figure this out sooner will operate more efficiently and can scale faster. Training staff to work effectively with AI, updating KPIs to measure AI-augmented processes, and addressing change management are all part of maintaining the moat. As noted, those who fall behind in adopting AI into their strategy may find it “hard to ever catch up”, because the leaders will be compounding advantages in data, efficiency and innovation speed year over year.
Ecosystem Collaboration vs. Competition
Finally, strategic positioning in the AI era involves choosing where to collaborate and where to compete. Open-source and shared research are hallmarks of AI development – contributing to or leveraging these can accelerate your own capabilities. Many startups build on open models (like LLaMA or open robot platforms) but add proprietary improvements. This collaborative approach can be smart: ride the wave of community advancement, but own the last-mile solution. Enterprises might partner with AI providers for general tools but keep their secret sauce (core algorithms or data) in-house. Those that manage this balance well – open where it benefits all, closed where it differentiates – will thrive in the emerging ecosystem.
Conclusion
The next 3–5 years will likely mark a pivotal transition in Web3 and AI: from the age of isolated chatbots to an era of agentic AI workflows and physically embodied intelligence. Technically, AI systems are becoming more autonomous, integrated, and capable of interacting with the real world. Market trends show accelerating adoption across enterprises and Web3 networks, though paced by considerations of ROI, regulation, and trust. Tremendous investment opportunities await in building the enabling layers and killer applications of this new AI paradigm – from agent platforms and robotics to AI-enhanced blockchain services and beyond.
For startups and enterprises: adapt and integrate AI pervasively or risk irrelevance. Those who harness AI to create ecosystem moats, optimize their workforce, and deliver superior experiences will lead their industries. Finally, the role of key enablers like NVIDIA cannot be overstated; their innovations in hardware and software will continue to flatten the path for AI developers and dramatically expand what’s possible.
For investors and builders in the Web3 and AI ecosystem: it’s a crucial time to participate in shaping this agentic, AI-driven future. If you are a founder building at the intersection of Web3 x AI, reach out to us at Decasonic - we are actively looking for founders innovating in this frontier.
The content of this material is strictly for informational and educational purposes only. It is not intended to constitute investment advice, nor should it be considered a recommendation or a solicitation to buy, sell, or hold any asset. Decasonic does not endorse investments in any specific tokens, and nothing in these blog posts should be construed as legal, tax, or financial advice. Please consult with a qualified professional advisor before making any financial decisions. Decasonic provides no warranties, whether expressed or implied, on the content provided in these blog posts, including its accuracy, completeness, or correctness. The opinions expressed here are those of the authors and do not necessarily reflect the views of Decasonic. Please note that Decasonic may hold a position in some of the tokens mentioned, including Virtuals. Decasonic is not liable for any errors or omissions in the content of this material or for any actions taken based on the information provided herein.
Comentarios